Imagine if AI could not only inform you that "the user is angry," but also pinpoint the reason as "due to latency and poor service”-this is the charm of self-explanatory models. However, existing methods often "fail" on pre-trained models. The PLMR method proposed in this paper enhances the reliability of AI explanations by pruning irrelevant information, helping you truly understand the user's heart.
Recently, the research achievement of our school's master student YUAN Libing (supervisor: Professor YU Kui), titled "Boosting Explainability through Selective Rationalization in Pre-trained Language Models," has been accepted by the top data mining conference KDD 2025, with Professor YU Kui as the corresponding author.
The ACM SIGKDD Conference on Knowledge Discovery and Data Mining (referred to as KDD), initiated in 1989, is the longest-standing and largest international top-tier academic conference in the field of data mining. KDD is also an A-category international conference recommended by the CCF China Computer Federation, ranking first in the academic rankings for data mining and analysis categories with an h5-index of 124.
Paper Title: Boosting Explainability through Selective Rationalization in Pre-trained Language Models
Authors: YUAN Libing, HU Shuaibo, YU Kui, WU Le
Paper Link: https://arxiv.org/html/2501.03182
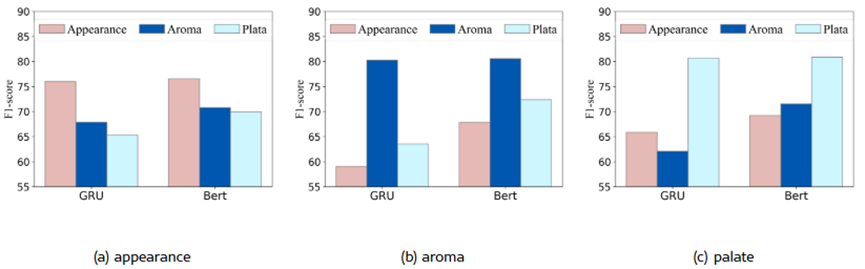
Figure 1.Comparison of spurious correlation strength in BERT and GRU representations
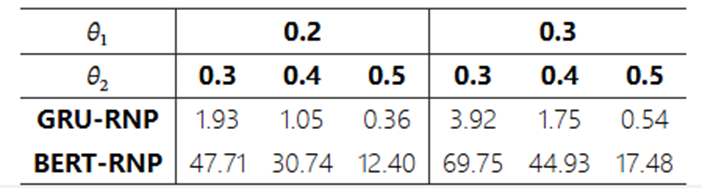
Table 1.Percentage of rationalization failure texts in the test set. Experiments on the aroma aspect of the BeerAdvocate
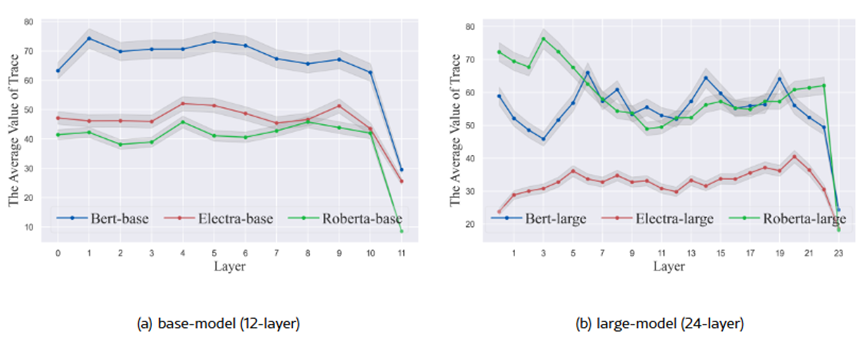
Figure 2. The average trace tr (Σ) of all sentences in different layers. Testing on the HotelReview dataset. The results are verified on three models: Bert, Electra, and Roberta

Figure 3.The proposed rationalization architecture PLMR. The dashed lines are used only during the training phase
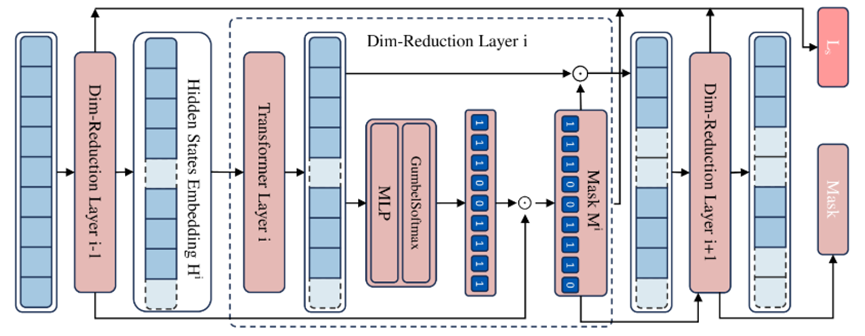
Figure 4. Detailed Design of the Dimension-Reduction Layer: The input is the hidden states H. The outputs are the final rationale mask M and the sum of all sparsity and continuity control losses
from the Dim-reduction layers
Abstract: The widespread application of pre-trained language models (PLMs) in natural language processing (NLP) has led to increasing concerns about their explainability. Selective rationalization is a self-explanatory framework that selects human-intelligible input subsets as rationales for predictions. Recent studies have shown that applying existing rationalization frameworks to PLMs will result in severe degeneration and failure problems, producing sub-optimal or meaningless rationales. Such failures severely damage trust in rationalization methods and constrain the application of rationalization techniques on PLMs. In this paper, we find that the homogeneity of tokens in the sentences produced by PLMs is the primary contributor to these problems. To address these challenges, we propose a method named Pre-trained Language Model’s Rationalization (PLMR), which splits PLMs into a generator and a predictor to deal with NLP tasks while providing interpretable rationales. The generator in PLMR also alleviates homogeneity by pruning irrelevant tokens, while the predictor uses full-text information to standardize predictions. Experiments conducted on two widely used datasets across multiple PLMs demonstrate the effectiveness of the proposed method PLMR in addressing the challenge of applying selective rationalization to PLMs. Codes: https://github.com/ylb777/PLMR.